Film Production Studios: Enhancing Audience Engagement and Content Creation
Gaining valuable insights to create more captivating and emotionally resonant content.
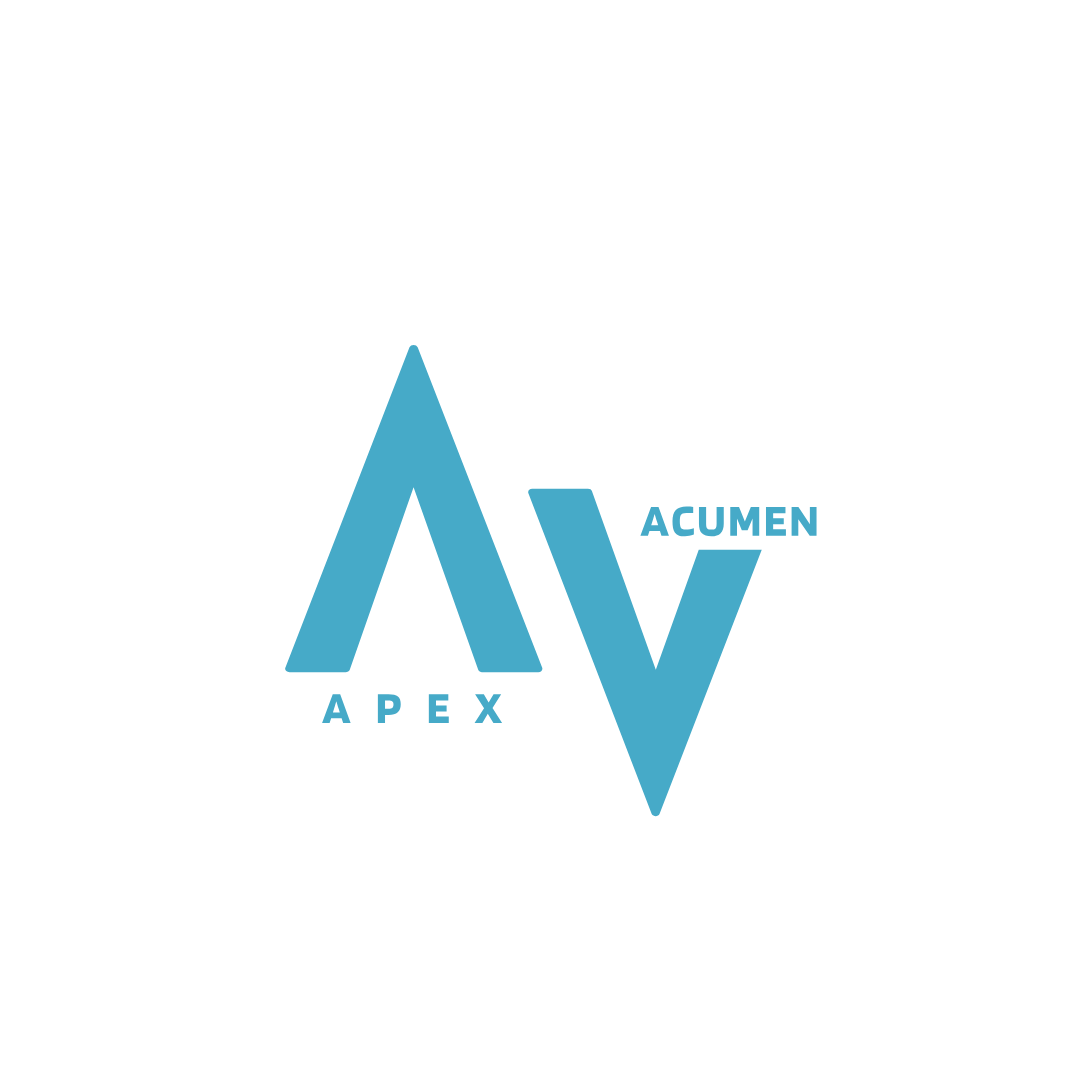
Gaining valuable insights to create more captivating and emotionally resonant content.
The film industry constantly evolves, with new technologies and techniques introduced to create more engaging and enjoyable content for audiences worldwide. One such technology is multimodal artificial intelligence (AI), which has the potential to revolutionize the way film production studios understand and cater to their audience. This article explores the use of multimodal AI in film production studios, focusing on its applications in emotion recognition, speech analysis, facial expression analysis, and action detection. It also highlights the benefits of using this technology in creating more engaging content and increasing customer satisfaction.
Multimodal AI uses multiple modalities, such as audio, visual, and textual data, to analyze and understand the context of a given situation 1. In film production, this technology can be applied to various aspects of content creation, from analyzing audience reactions to optimizing the storytelling process. Let's discuss how multimodal AI can enhance the film production process and create a more engaging and immersive experience for viewers.
One of the most vital elements of storytelling is the ability to evoke emotions in the audience. By using multimodal AI algorithms, film production studios can analyze the emotions of their viewers, allowing them to understand which scenes were most emotionally impactful 3. This information can then be used to refine the narrative and ensure the story resonates with the target audience. For example, if a scene does not evoke the desired emotional response, the filmmakers can adjust to achieve their intended effect better.
Another application of multimodal AI in film production is the analysis of speech patterns. By analyzing characters' dialogue and vocal intonation, AI algorithms can provide insights into the script's effectiveness in conveying the intended emotions and messages 2. This can help screenwriters and directors make informed decisions on adjusting the script or directing the actors to deliver lines more effectively.
Facial expressions are a crucial aspect of non-verbal communication in films and an essential component of an actor's performance. Multimodal AI can analyze facial expressions, providing feedback on the effectiveness of an actor's portrayal of a character's emotions 5. This information can be valuable for the actors and the director, helping them fine-tune their performances to better connect with the audience.
In addition to emotion recognition and facial expression analysis, multimodal AI can also be used to analyze the actions and movements of characters within a film. This can help filmmakers identify trends or patterns in the audience's engagement and preferences, allowing them to create more compelling and dynamic scenes 4. For example, understanding which action sequences resonate with viewers can help filmmakers craft more exciting and visually appealing content.